As an industry, the importance of providing in-depth coverage on a topic is essential for brand relevance and ranking. With Google’s helpful content updates, there have been cases of companies experiencing reduced rankings and traffic because they employed a spray-and-pray approach. That is employing a content marketing approach that involves creating articles for high search volume topics for the sake of generating website visits. A traffic-first approach that does not cater to the user’s needs and fails to build a solid depth or authority in a particular niche topic. With E-E-A-T (Experience, Expertise, Authoritativeness and Trustworthiness), there is no room for a blanket content marketing approach. Companies have to build an authority in a given topic that is closely related to their brand, product or service. But the journey does not need to stop there, more concepts need to be developed to help us better understand the intent of searchers and analyse how the available content is catering to these needs. For this, I am proposing the concept of ‘Semantic Authority’, an evolution of topical authority. This concept will be introduced below.
What is Semantic Authority
Semantic authority is the degree to which a website demonstrates a contextual, cognitive, and causal understanding of a domain by aligning with user intent, interpreting meaning accurately, and structuring information relationally and dynamically. To put this simply, semantic authority involves a more contextual and cognitive understanding of a topic or concept and developing the required content to cater to the user’s needs. Websites that have fully broken down the semantic relations of a concept or seed term are more likely to create contents that go a step above topical coverage. These brands and websites can be viewed to attain an authority in the meaning or semantic space.
Read more: Introducing the concept of semantic authority in search marketingHow Semantic Authority is an Evolution of Topical Authority
Semantic authority adds the contextual, cognitive and causal layer to topical authority. It is important to revisit the meaning of topical authority. There is a great blog on the Ahrefs website about topical authority. Reminding us all about how it is geared towards creating sufficient content to cover all relevant keywords for a given topic. The example used in the Ahrefs blog was for a seed term “protein powder,” and the examples provided in the same blog added that every aspect of protein powder should be covered by a website like the one below if they plan to build a topical authority.
- “what is protein?”
- “what does protein powder do”
- “what is the best protein powder”
- “how to use protein powder”
- “how long does protein powder last”
- “how to use protein powder for weight loss”
This makes perfect sense as it seeks out a keyword-first approach to build a topical cluster that sets the basis for the creation of content to fully establish topical authority. There is a section in the blog that talks about how to build topical authority, and it was suggested that the first step was to conduct keyword research to find all the talking points within a topic. I will argue that a semantic authority approach focuses on breaking down a concept, seed term, or a topic to possibly how users see it and then run keyword research based on the outline, concept anatomy or structure. To achieve this, a cognitive-first approach or a part-whole relations common in categorisation theory can be employed to analyse the seed term or concept of ‘Protein Powder’ based on its form, function and engagement. Utilising a semantic knowledge base such as ConceptNet can provide prescribed relations that can create the appropriate basis for a more in-depth and deeper keyword research exercise to be carried out.
Let’s look at how the seed term ‘Protein Powder’ can be broken down into relevant aspects and segmented based on the form, function and interaction aspects of the part-whole relations used in cognition.
With the help of an LLM like ChatGPT, I was able to identify relevant relations and terms in a human-friendly way. This can then be expanded with an in-depth keyword research process.
From the above, one can determine how semantic authority goes beyond a hierarchical or pyramid style to a more graph-like, semantically rich and sequential process. This is an introduction to the concept of semantic authority. More blogs will be written to deconstruct and analyse this concept and related ideas more effectively.
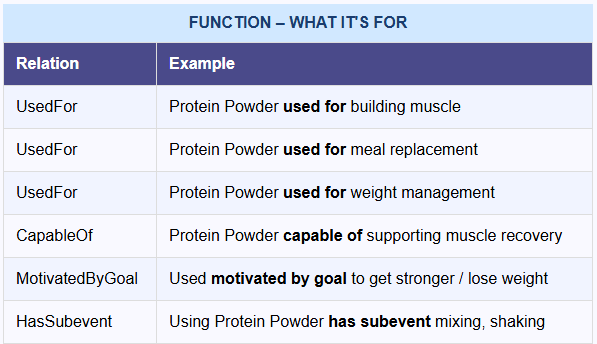
This is an example of how semantic authority can be achieved via a deep contextual and cognitive approach rather than a text or lexical similarity shared on the blog example from Ahrefs.
This is just scratching the surface of why Semantic authority is required as an evolution of topical authority, as the former tries to approach seed terms, concepts or topics from the user’s perspective, and the latter tends to default to start with keyword research. Search volumes may not always reflect how people view these concepts, like “protein powder.” And the meaning people hold of these concepts tends to evolve, and this may not be reflected in keyword research volumes.
Human behaviour and user needs are dynamic. A static content structure is not always sufficient; hence, the need to continuously assess the context. That’s why semantic authority is required to evaluate how brand content captures the temporal and evolving meaning of concepts or seed terms.